The Evolution and Future of AML Compliance Technology
Anti-Money Laundering (AML) compliance technology has experienced a significant transformation over the past few decades. Initially driven by regulatory requirements, the field has evolved into a dynamic area of innovation, leveraging cutting-edge technologies like artificial intelligence (AI), machine learning (ML), and real-time data analytics. As financial institutions worldwide contend with increasingly sophisticated financial crimes, AML compliance technology must continuously adapt and improve. This article explores the history, current state, and future trends of AML compliance technology, highlighting how Vneuron is leading the charge in this critical field.
The History of AML Compliance Technology
Early Beginnings: The Regulatory Push
AML compliance technology’s roots can be traced back to the regulatory frameworks established to combat money laundering. The Financial Action Task Force (FATF), established in 1989, played a pivotal role in setting international standards for AML. Initial AML systems were primarily rule-based, relying on predefined criteria to flag suspicious activities. These early systems, although groundbreaking at the time, were limited in their ability to detect sophisticated laundering schemes.
What are Rule-Based Systems?
Rule-based systems were the first generation of AML compliance technology. These systems operated on a set of predefined rules and thresholds to detect suspicious activities. For example, a transaction exceeding a certain amount or a series of transactions within a short period could trigger an alert. While rule-based systems were effective in identifying straightforward suspicious patterns, they struggled with more complex and evolving money laundering techniques.
The 1990s: The Advent of Transaction Monitoring Systems
In the 1990s, the introduction of automated transaction monitoring systems marked a significant leap in AML compliance technology. These systems were designed to analyze large volumes of transactional data to identify patterns indicative of money laundering. While these systems improved detection capabilities, they often generated a high number of false positives, leading to inefficiencies in the compliance process.
How Was The Early Transaction Monitoring
Early transaction monitoring systems relied heavily on static rules and thresholds. Financial institutions had to manually update these rules based on new regulatory requirements or emerging money laundering tactics. This manual process was labor-intensive and often led to delayed detection of suspicious activities. Moreover, the high rate of false positives required extensive human intervention to investigate and resolve alerts, placing a considerable burden on compliance teams.
The 2000s: The Rise of Risk-Based Approaches
The early 2000s saw a shift towards risk-based approaches in AML compliance. Financial institutions began to tailor their AML efforts based on the risk profiles of their customers and transactions. This period also witnessed the integration of Know Your Customer (KYC) processes with AML systems, enhancing the ability to monitor and assess customer behavior over time.
What Is a Risk-Based AML Methodology
Risk-based AML approaches prioritize resources toward higher-risk customers and transactions. This methodology involves assessing the risk level of each customer based on various factors such as geographic location, transaction history, and customer type. By focusing on higher-risk areas, financial institutions can allocate their resources more effectively and improve the efficiency of their AML efforts.
The 2010s: Big Data and Machine Learning
The explosion of big data and advances in machine learning during the 2010s revolutionized AML compliance technology. Machine learning algorithms enabled systems to learn from historical data and improve their detection capabilities over time. These technologies significantly reduced false positives and enhanced the ability to identify complex money laundering schemes. Additionally, the use of big data allowed for more comprehensive analysis of customer and transactional data, leading to more accurate risk assessments.
Machine Learning in AML
Machine learning (ML) brought a paradigm shift in AML compliance technology. Unlike rule-based systems, ML algorithms can learn and adapt to new patterns without explicit programming. These algorithms analyze historical transaction data to identify anomalies and predict future suspicious activities. The ability to learn from data and adapt to new threats made ML-based systems more effective in detecting sophisticated money laundering schemes.
Current State of AML Compliance Technology
1. Integration of Artificial Intelligence
Today, AI is at the heart of modern AML compliance technology. AI-powered systems can process vast amounts of data in real-time, identifying suspicious activities with unprecedented accuracy. These systems utilize various AI techniques, including natural language processing (NLP) for analyzing unstructured data, anomaly detection algorithms for identifying unusual patterns, and predictive analytics for anticipating future risks.
● Natural Language Processing
NLP enables AML systems to analyze unstructured data such as text documents, emails, and social media posts. By understanding and extracting relevant information from these sources, NLP enhances the ability to identify suspicious activities that may not be evident from structured transactional data alone. For example, NLP can detect mentions of illegal activities or adverse media reports related to a customer.
● Anomaly Detection
Anomaly detection algorithms identify deviations from normal behavior that could indicate suspicious activities. These algorithms learn the typical patterns of transactions and flag any deviations for further investigation. For instance, a sudden increase in transaction volume or frequency for a particular customer may trigger an alert.
● Predictive Analytics
Predictive analytics involves using historical data to predict future suspicious activities. By identifying patterns and trends in past transactions, predictive models can anticipate potential money laundering schemes before they occur. This proactive approach allows financial institutions to mitigate risks more effectively.
2. Advanced Transaction Monitoring
Advanced transaction monitoring systems now incorporate sophisticated analytics to detect suspicious activities, leveraging machine learning models trained on historical data to recognize complex laundering patterns that rule-based systems would miss. Real-time monitoring capabilities analyze data as it flows through financial networks, enabling immediate detection and response to potential threats.
According to observations from Vneuron’s clients, those who have implemented advanced transaction monitoring systems have reported a more than 50% reduction in false positives and approximately a 70% improvement in detecting suspicious activities compared to traditional systems. This real-time analysis and alert system allow compliance teams to investigate and respond to potential threats immediately, reducing the risk of financial crimes.
3. Comprehensive KYC and EDD Solutions
Comprehensive KYC and Enhanced Due Diligence processes have seen remarkable advancements due to technological innovations. Modern solutions utilize AI and machine learning (ML) algorithms to analyze a wide array of data sources—including social media, news articles, and public records—to construct detailed profiles of high-risk customers. This comprehensive approach enables financial institutions to more effectively assess and manage the risks associated with their clients.
● Advanced KYC Processes
KYC procedures are essential for verifying customer identities and evaluating potential risks tied to business relationships. Advanced KYC solutions integrate multiple data sources to create thorough customer profiles. These profiles include a variety of information, such as identification details, transaction histories, business affiliations, and potential risk factors. By leveraging AI and ML technologies, these solutions automate data collection and analysis, reducing the need for manual processing and streamlining the KYC process.
● Enhanced Due Diligence (EDD)
EDD processes are vital for assessing high-risk customers. AI and ML algorithms allow financial institutions to perform deeper analyses of customer data, uncovering risk factors that traditional methods might miss. Modern EDD solutions aggregate and scrutinize data from diverse sources, providing an in-depth view of customer activities and behaviors. This advanced analysis helps institutions proactively identify and mitigate risks, enhancing their overall risk management and compliance efforts.
4. Screening and Real-Time Capabilities
Screening for sanctions, politically exposed persons (PEPs), and adverse media has been greatly enhanced by AI-driven technologies, which ensure real-time capabilities for immediate integration of new information into risk assessments.
This advancement is crucial for AML compliance, as it allows financial institutions to continuously update sanctions lists and PEP databases, thereby maintaining compliance with the latest regulatory requirements and minimizing the risk of financial crimes.
AI-driven screening solutions provide a dynamic approach to monitoring transactions and customers, ensuring that institutions avoid engagements with sanctioned entities and individuals while staying abreast of evolving regulatory landscapes.
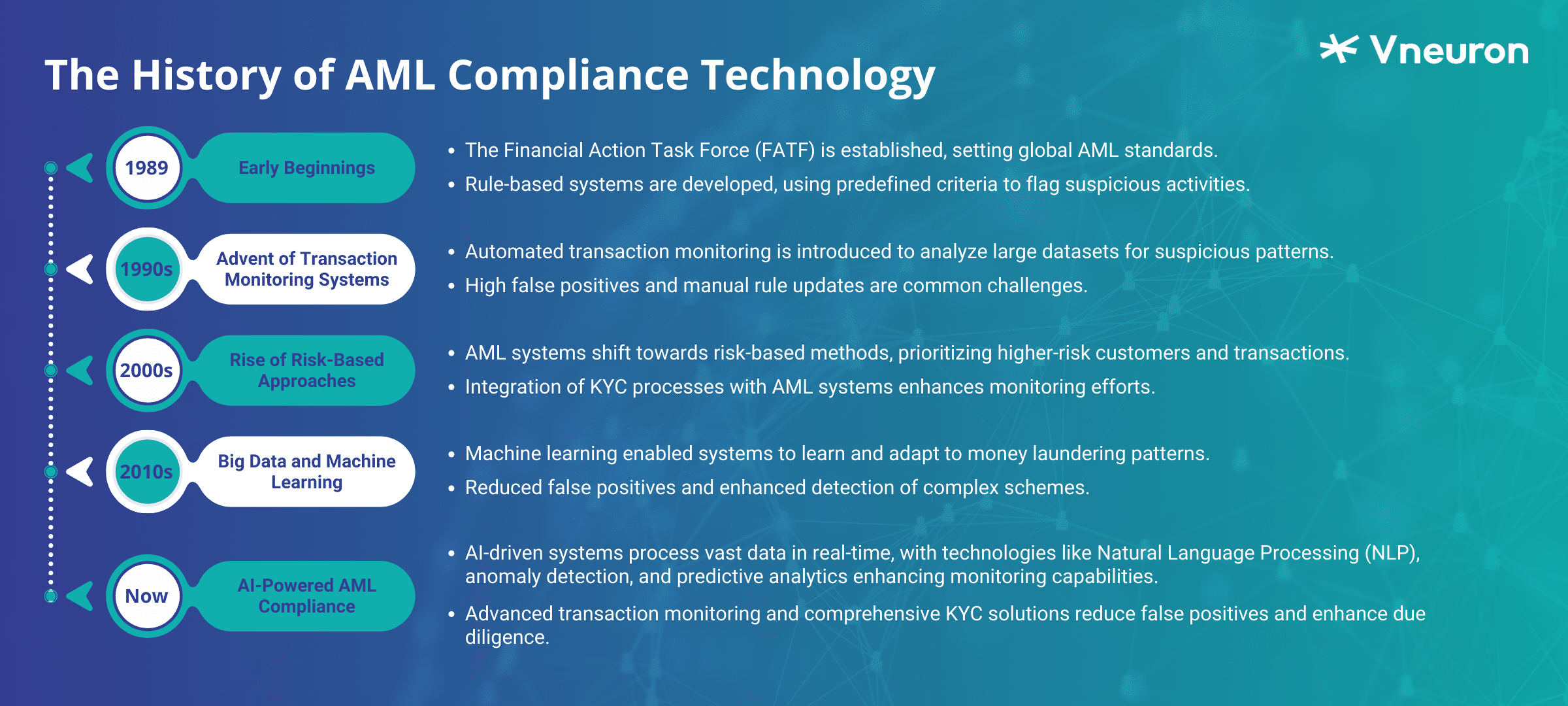
Vneuron's Role in Leading Innovation
- Pioneering AI-Driven AML Solutions: Vneuron leads the field with its AI-driven AML solutions, featuring the Reis™ Risk & Compliance Suite that integrates advanced AI algorithms for real-time monitoring and risk assessment. The system continuously learns from new data, adapts to emerging threats, and minimizes false positives, allowing compliance teams to focus on genuine risks.
- Continuous Learning and Adaptation: AI-driven AML solutions offer continuous learning and adaptation, unlike traditional rule-based systems that require manual updates. AI algorithms evolve by learning from new data, keeping the system ahead of emerging threats and evolving money laundering techniques.
- Comprehensive KYC and EDD Solutions: Beyond traditional transaction monitoring, Vneuron’s technology provides comprehensive KYC and EDD capabilities. By aggregating data from diverse sources, it delivers a 360-degree view of customers, enhancing compliance and supporting better organizational decision-making.
- Data Aggregation and Analysis: The KYC and EDD solutions utilize AI and ML to aggregate and analyze data from multiple sources, offering a holistic view of customers’ risk profiles. This approach automates data collection and analysis, reducing the burden on compliance teams and improving efficiency.
- Advanced Screening Technologies: Vneuron’s advanced screening tools use AI for real-time screening of sanctions, PEPs, and adverse media. This ensures financial institutions stay compliant with regulatory requirements and adapt swiftly to new risks.
- Real-Time Screening and Alerts: Real-time screening is crucial for maintaining compliance. Vneuron’s technologies continuously update sanctions lists and PEP databases, providing the latest information. Real-time alerts facilitate immediate investigation of potential risks, reducing the likelihood of financial crimes and regulatory fines.
Future Trends in AML Compliance Technology
1. Greater Integration of AI and ML
The future of AML compliance technology will be marked by an even deeper integration of AI and ML, driving significant advancements in algorithm sophistication. As these technologies evolve, future AML systems will feature highly advanced algorithms capable of analyzing larger volumes of data and identifying intricate patterns with greater accuracy. By leveraging deep learning techniques, these systems will detect subtle anomalies and predict future suspicious activities.
Continuous learning and adaptation will be essential, ensuring that these solutions remain effective in addressing evolving money laundering threats. Moreover, these systems will incorporate more complex models capable of recognizing sophisticated laundering techniques that were previously undetectable, enhancing overall security and compliance.
2. Expansion of Real-Time Monitoring
Real-time monitoring will become even more critical as financial transactions become faster and more complex. Future AML systems will need to analyze data instantaneously, providing immediate alerts and enabling rapid responses. Advancements in data processing capabilities will enable future AML systems to analyze data in real-time, even as transaction volumes continue to increase. High-performance computing and distributed processing technologies will play a crucial role in achieving this real-time capability. Financial institutions will be able to detect and respond to suspicious activities immediately, reducing the risk of financial crimes. This shift towards real-time monitoring will also involve the development of more efficient algorithms that can handle the increased data flow without compromising on speed or accuracy.
3. Enhanced Collaboration and Data Sharing
Enhanced collaboration and data sharing will be pivotal for the future of AML compliance, especially with the growing trend of open finance. As financial institutions, regulators, and technology providers increasingly work together, shared databases and collaborative platforms will become essential. These platforms will aggregate data from multiple sources, enabling more comprehensive analysis and effective detection of cross-institutional money laundering schemes. With the rise of open finance, which promotes greater data accessibility and integration across institutions, privacy-preserving technologies such as secure multi-party computation and federated learning will be critical to ensuring secure and compliant data sharing while protecting customer information. This collaborative approach will not only improve detection rates but also foster a more unified and proactive stance against financial crimes.
4. Emphasis on Explainability and Transparency
As AI and ML systems become increasingly integral to AML compliance, the emphasis on explainability and transparency will intensify. Regulators and financial institutions will demand clear, understandable explanations of how these systems make decisions to adhere to legal and ethical standards. This focus will drive the development of AI models that are not only effective but also interpretable.
Explainable AI (XAI) will become a key component of future AML solutions, providing transparent insights into how AI algorithms identify suspicious activities. By incorporating XAI techniques, these solutions will facilitate greater trust and confidence among compliance teams and regulators, ensuring alignment with regulatory requirements and ethical guidelines. Transparency will also help in educating stakeholders about the capabilities and limitations of AI, promoting more informed decision-making.
5. Integration of Blockchain Technology
Future AML compliance will increasingly integrate blockchain technology to enhance data integrity and transparency. Blockchain’s immutable ledger and decentralized nature will improve the accuracy and reliability of transaction records, making it easier to trace and verify transactions.
This integration will help combat money laundering by providing a clear and tamper-proof audit trail, thereby reducing the risk of fraudulent activities and improving overall compliance. Blockchain technology will also facilitate better record-keeping and auditing processes, enabling quicker and more accurate investigations into suspicious transactions.
6. Increased Use of Behavioral Analytics
Behavioral analytics will become a more prominent trend in AML compliance, focusing on understanding and predicting customer behavior patterns. Future AML systems will leverage advanced behavioral analytics to identify deviations from typical behaviors and detect potentially suspicious activities. By analyzing behavioral trends and anomalies, these systems will enhance the accuracy of risk assessments and improve the ability to preemptively address emerging money laundering schemes.
Behavioral analytics will provide deeper insights into customer activities, allowing financial institutions to implement more personalized and effective compliance measures.
The evolution of AML compliance technology reflects broader trends in the financial industry towards greater sophistication, integration, and real-time capabilities. From the early rule-based systems to today’s AI-driven solutions, the field has made significant strides in combating money laundering. As we look to the future, continued innovation in AI, real-time monitoring, and collaborative platforms will be essential to stay ahead of increasingly sophisticated financial crimes.
Financial institutions must embrace these advancements to protect themselves and the broader financial system. The future promises even more exciting developments, and staying at the forefront of technological advancements will ensure that they are equipped with the most effective tools to combat money laundering and comply with regulatory requirements. The commitment to driving progress in AML compliance technology is vital for maintaining the integrity of financial systems worldwide.
Stay ahead of regulatory demands and sophisticated financial crimes with Vneuron’s innovative solutions. Contact us today to learn more.